Date: 2023
Duration: 3 weeks
Instructor: Kivanc Kose, Bilge Kose
Roal: Research, Data Labeling, Programming (Based on provided code)
Background
The project focuses on three specific historic districts in New York: Prospect Park South, Ditmars Park, and Fiske Terrace-Midwood. There is an increasing need to incorporate sustainable energy solutions, such as solar panels, in these historic districts. However, it is important to integrate these solutions in a way that respects the architectural integrity and historical value of the areas. For instance, solar panels should be installed on the secondary facade of the building, away from the main road.
Therefore, it is worth discussing how to ensure that solar panels on the roof of a secondary facade receive maximum sunlight.
Project Objective
Develop an efficient AI-based plan for installing solar panels in the historic districts of New York that complies with LPC regulations.
From Satellite Images to Data Labeling Annotation
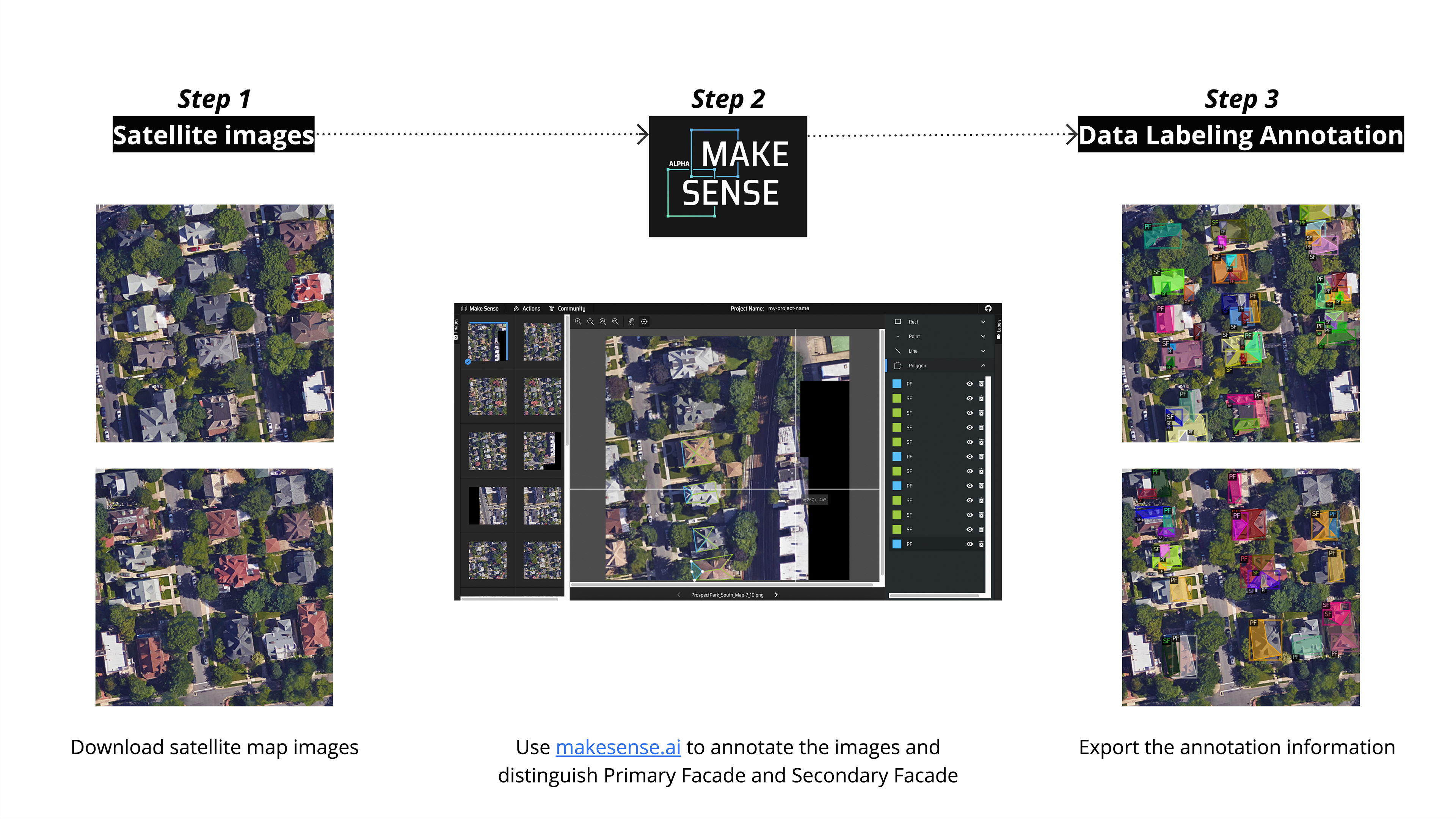
Data Labeling Annotation in Makesense.Ai
Application of Object Detection Model
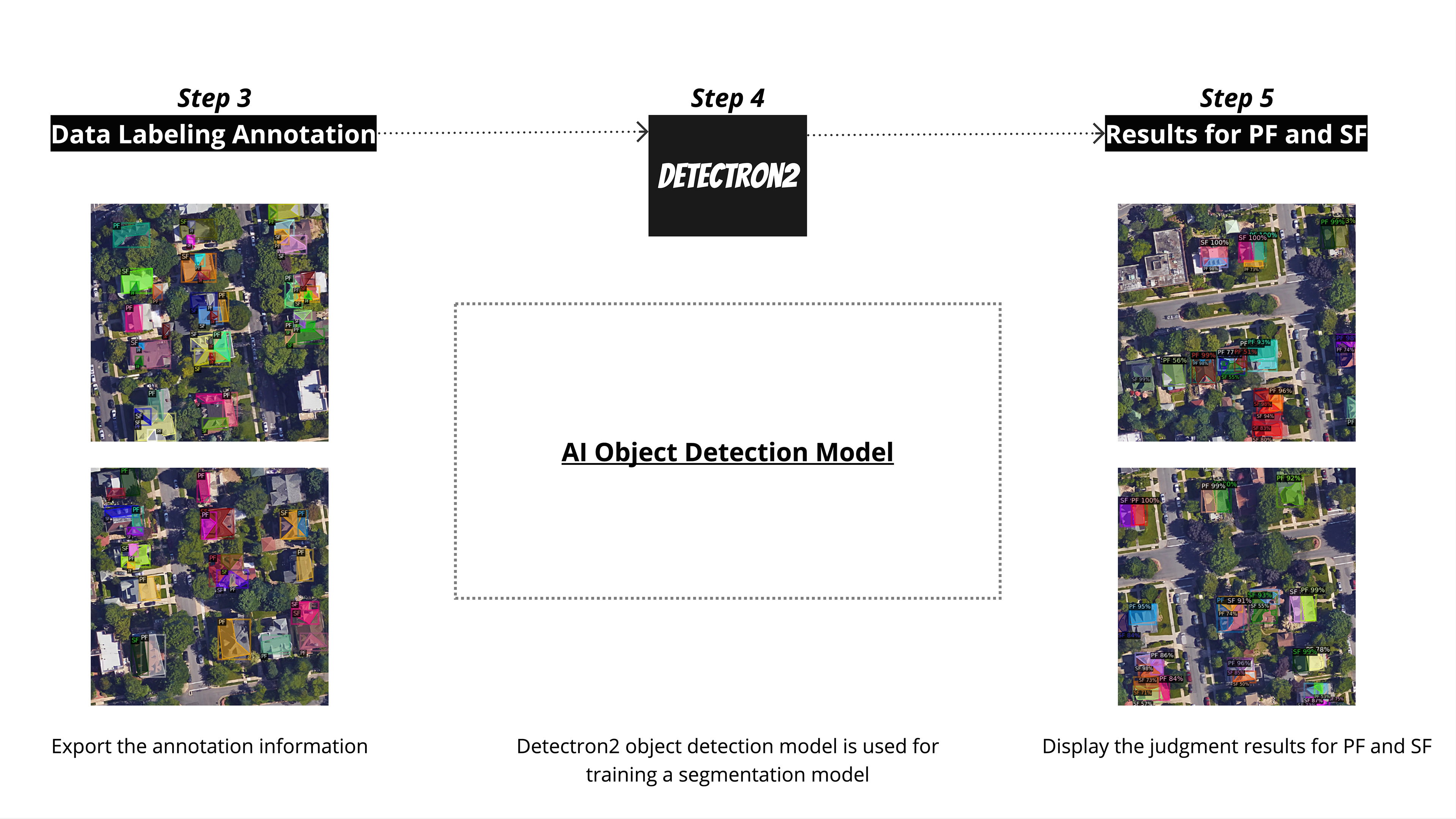
Combination of Google Maps & Google Solar API
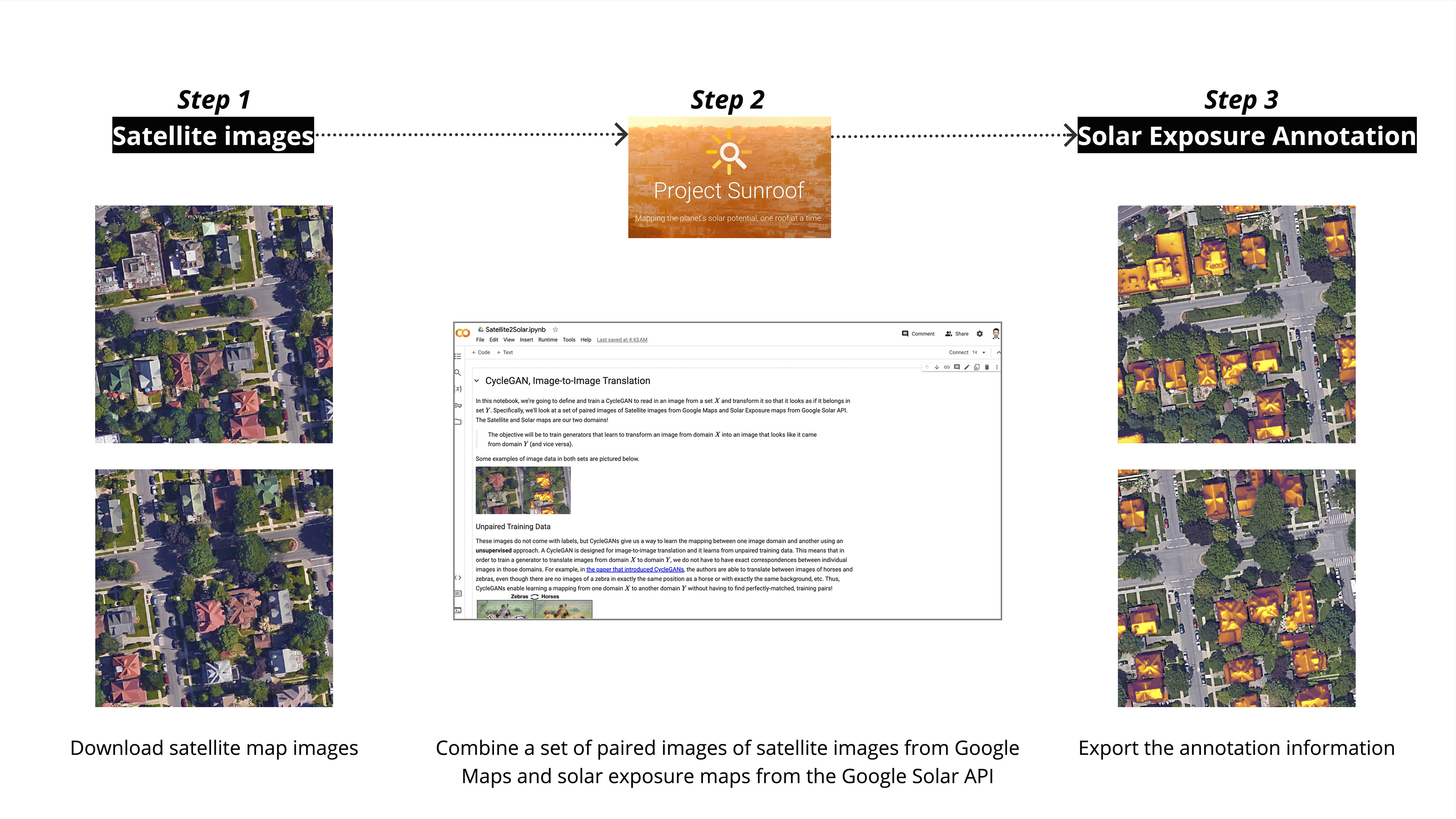
Overlay Secondary Facade & Solar Exposure Map
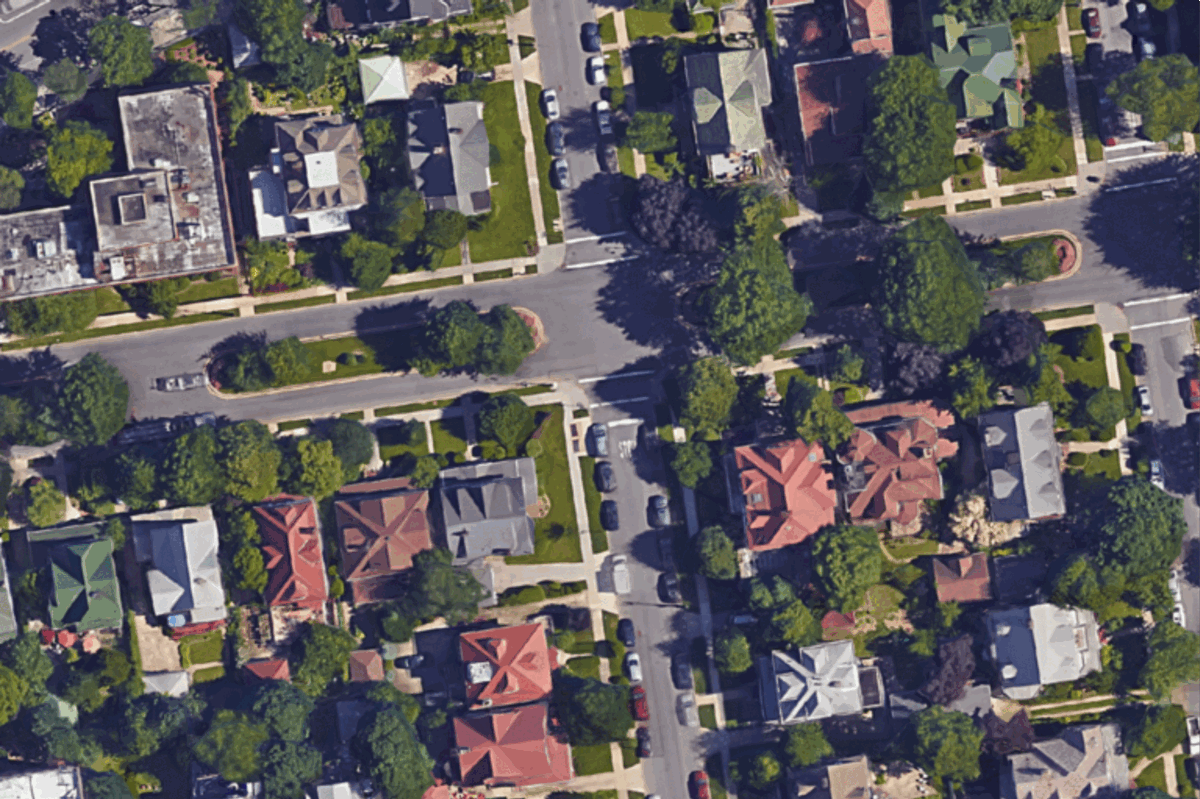
Potential Benefits for Stakeholders
Benefits for LPC:
Ensuring that the solar panel installation plan meets historic preservation standards while promoting the use of sustainable energy.
Benefits for Property Owners:
Enhancing energy efficiency and reducing long-term energy costs.
Benefits for Architectural Firms:
Offering efficient design solutions, thereby reducing design time and costs.
Next Steps for the Project
Expand Model Training:
Incorporate more data from historic districts to improve the accuracy and applicability of the model.
Field Testing:
Implement pilot projects in selected districts to assess the model's performance in real-world environments.
Advantages of AI over Manual Work
Enhanced Efficiency:
Swift analysis of large datasets to provide real-time design solutions.
Improved Accuracy:
Ability to identify suitable areas for solar panel installation with greater precision.
Cost Savings: Reduction in on-site surveying manpower costs.
Limitations
Data Dependency:
Currently, the training dataset consists of only 129 images, which is limited in size.
Scalability:
The model may require adjustments to accommodate different architectural styles and environmental conditions in various districts.